Bootstrap In Finance
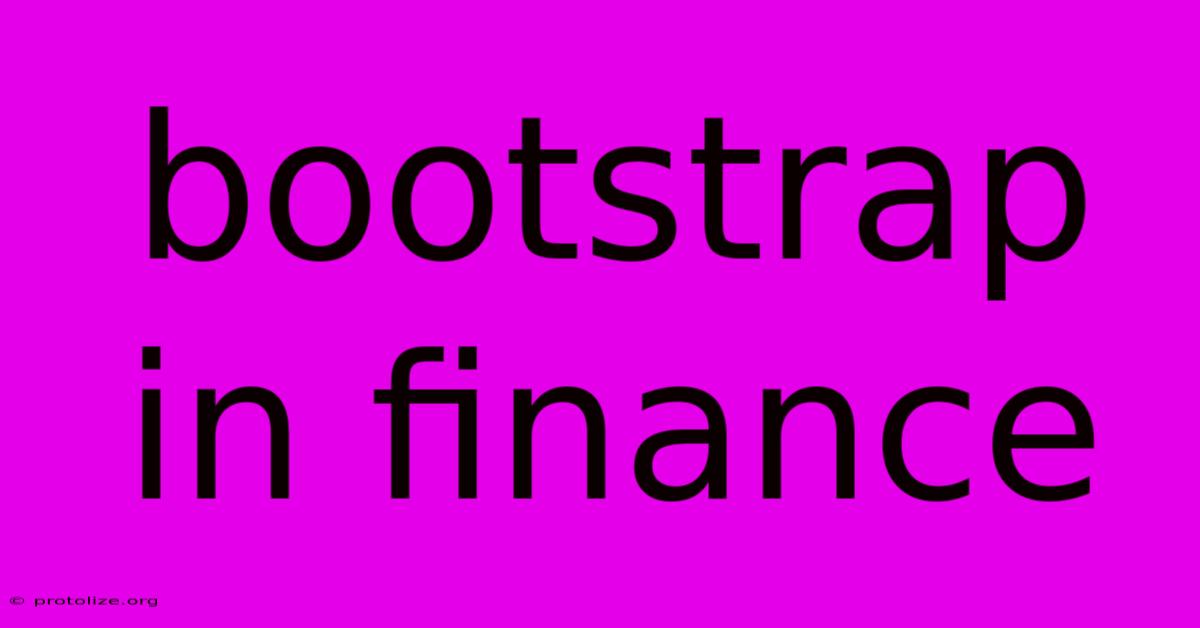
Discover more detailed and exciting information on our website. Click the link below to start your adventure: Visit Best Website mr.cleine.com. Don't miss out!
Table of Contents
Bootstrap in Finance: A Powerful Tool for Financial Modeling and Analysis
The term "bootstrap" might conjure images of pulling oneself up by one's bootstraps β a feat of incredible self-reliance. In the world of finance, bootstrapping takes on a similar connotation, representing a powerful technique for estimating statistical properties and creating robust financial models. This article dives into the multifaceted applications of bootstrapping in finance, exploring its advantages and limitations.
What is Bootstrapping in Finance?
Bootstrapping is a resampling technique used to estimate the sampling distribution of a statistic. In simpler terms, it allows financial analysts to simulate multiple scenarios based on existing data, providing a clearer picture of potential outcomes and uncertainties. Instead of relying on theoretical assumptions about data distribution (which may not always hold true), bootstrapping uses the observed data itself to generate a range of possible results. This makes it particularly useful when dealing with complex financial datasets that defy traditional statistical approaches.
How Does Bootstrapping Work?
The process is surprisingly straightforward:
-
Sampling with Replacement: A random sample of the same size as the original dataset is drawn from the original dataset with replacement. This means that some data points might be selected multiple times, while others might be omitted entirely.
-
Statistic Calculation: The chosen statistic (e.g., mean, standard deviation, correlation coefficient) is calculated for this resampled dataset.
-
Iteration: Steps 1 and 2 are repeated a large number of times (e.g., 1000 or more) to create a distribution of the statistic.
-
Inference: This distribution of statistics provides insights into the variability and uncertainty associated with the original estimate. Confidence intervals can be constructed to quantify this uncertainty.
Applications of Bootstrapping in Finance
Bootstrapping finds numerous applications in various areas of finance, including:
1. Risk Management:
- Value at Risk (VaR) Estimation: Bootstrapping helps to estimate VaR more accurately, particularly when dealing with non-normal returns. By resampling historical returns, analysts can generate a distribution of potential portfolio losses and quantify the risk more precisely.
- Stress Testing: Bootstrapping can simulate various stress scenarios by resampling data under different market conditions, allowing for a more robust assessment of risk under extreme events.
2. Portfolio Management:
- Performance Attribution: Bootstrapping can help disentangle the various factors contributing to portfolio performance, giving a clearer understanding of managerial skill versus market movements.
- Optimal Portfolio Construction: By generating multiple portfolio scenarios based on bootstrapped data, investors can explore a wider range of possible portfolios and identify optimal allocations based on risk and return objectives.
3. Derivatives Pricing:
- Estimating Volatility: Bootstrapping can be used to estimate volatility more accurately, a critical parameter in options pricing models. The bootstrapped volatility distribution accounts for the uncertainty inherent in volatility forecasts.
4. Credit Risk Modeling:
- Default Probability Estimation: Bootstrapping can improve the accuracy of default probability estimates for loans and bonds by accounting for the inherent uncertainty in the historical default data.
Advantages of Bootstrapping
- Non-parametric: Bootstrapping doesn't rely on assumptions about the underlying data distribution, making it suitable for complex, non-normal data often encountered in finance.
- Flexibility: It can be applied to a wide range of statistics and datasets.
- Intuitive: The method is relatively easy to understand and implement.
- Improved Accuracy: It often leads to more accurate estimations compared to traditional parametric methods, especially when sample sizes are small or data distributions are unknown.
Limitations of Bootstrapping
- Computational Intensity: Bootstrapping can be computationally intensive, particularly when dealing with large datasets or complex models.
- Bias: In some cases, bootstrapping can introduce bias if the original sample is not representative of the population.
- Interpretation: Interpreting the results of bootstrapping requires a solid understanding of statistical concepts.
Conclusion
Bootstrapping has emerged as a valuable tool in the financial analyst's toolkit. Its ability to handle complex data and provide robust estimations of uncertainty makes it particularly well-suited for tackling the challenges of financial modeling and analysis. While computational limitations exist, the advantages often outweigh the drawbacks, making bootstrapping a powerful method for improving decision-making in various aspects of finance. As computational power continues to grow, expect to see even broader adoption of this versatile resampling technique in the years to come.
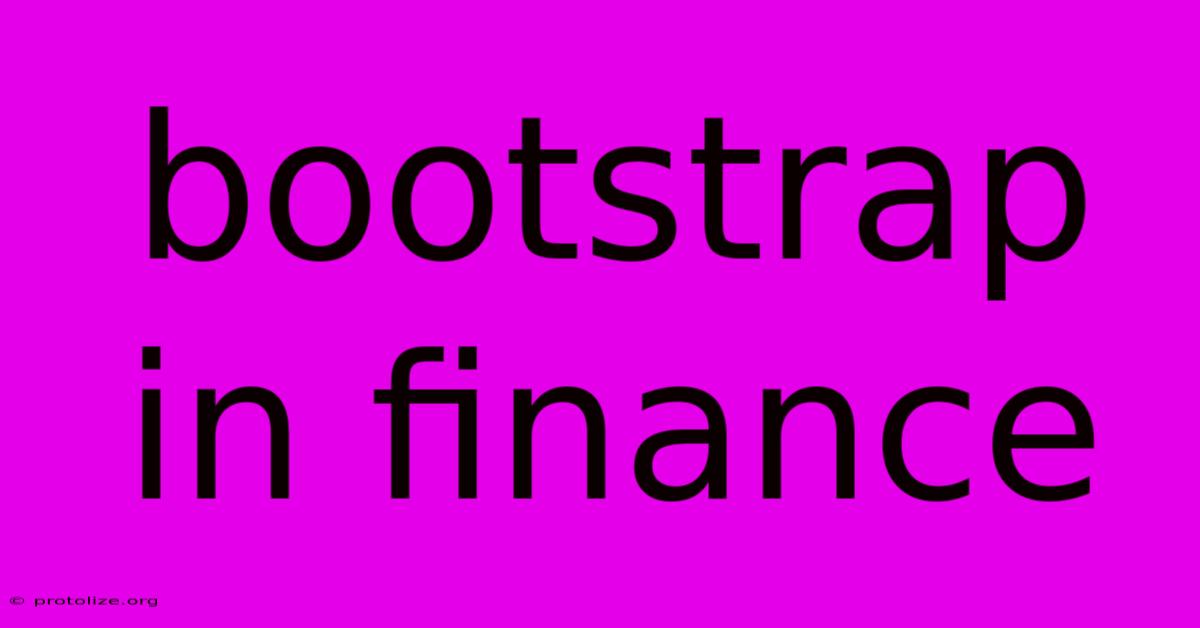
Thank you for visiting our website wich cover about Bootstrap In Finance. We hope the information provided has been useful to you. Feel free to contact us if you have any questions or need further assistance. See you next time and dont miss to bookmark.
Featured Posts
-
Finance Tips For Women
Dec 16, 2024
-
Investments In Finance
Dec 16, 2024
-
Best Practice Finance Department Structure
Dec 16, 2024
-
Finance Life Insurance Premium
Dec 16, 2024
-
Bus Finance
Dec 16, 2024